Bayesian networks, or Bayesian belief networks (BBN), are directed graphs with probability tables, where the nodes represent relevant variable dependencies that can be continuous or discrete. The arcs represent causal relationships between a variable and outcome. As an example, an input such as “weather” could affect how one drives their car. The intent of such networks is to understand events that have a degree of uncertainty. In effect, they look at probabilistic events, where variables may define if something could occur and what those relationships are that can cause something to occur (e.g., a car accident in the example given).[1]
Using Bayesian Belief Networks and GIS as Decision-Making Aides
While BBNs can be used within GIS, researchers have focused on defining spatially probabilistic models, where BBNs can help define relevant spatial relationships. Typically, BBNs have been used in ecological studies or environmental management. For instance, in understanding social-environmental interactions, where a complex set of variables could affect outcomes of the environment and subsequent human decisions in landscape/ecological management, BBNs and GIS can be used as decision aides to give an idea of probability of events unfolding. They assist decision-making by also defining key variables and how they could affect outcomes.[2]
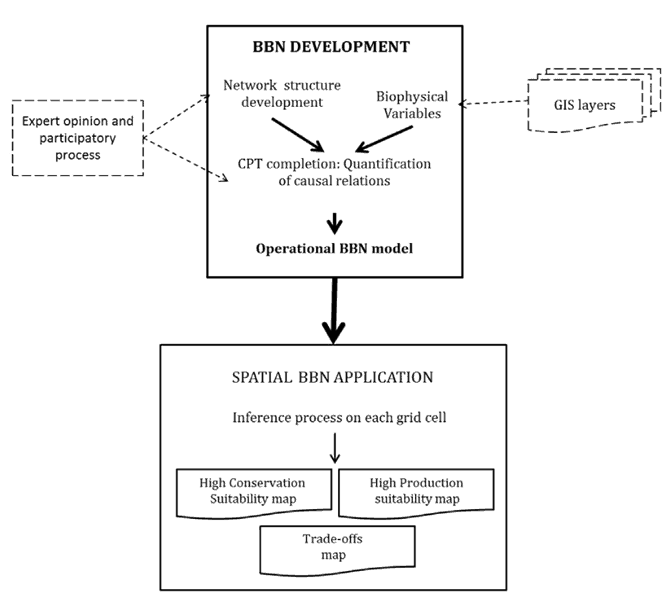
Applications of Bayesian Belief Networks
Common applications include modeling scenarios of BBNs so that likelihood of event outcomes could be better determined. For instance, if wildlife managers wanted to determine if a particular conservation plan might work, then utilizing the set of variables for given species in a new location and environment could be modeled and assessed prior to the implementation of the conservation plan. This allows managers to get an idea of potential impacts on the habitat and species prior based on known species behavior and environmental relationships. This was the case, as an example, for the Australian Julia Creek dunnart, where conservation efforts looked at the species.[3] Additionally, BBNs have been applied for assessing the prioritization and licensing of hydropower licenses, including where decision criteria can affect how hydropower is licensed and environmental-related impacts this might create.[4] The critique in environmental related research, in particular ecosystems research, has been there has been primarily a focus on a single system rather than looking at larger, integrated systems. Furthermore, feedback loops and relationships that are potential more complex are often not assessed in the literature.[5]
While environmental-related studies have largely dominated the use of BBNs, other recent areas of interest including studying infrastructure-related problems. For instance, a probabilistic modeling effort using BBNs was applied in studying risk to water main failure. The idea is to use historical and spatial data to see which water mains could likely rupture or create problems, and thus forecast also possible budgets and likely costs, for a given water infrastructure network. This modeling approach can help communities and local councils better manage their water distribution systems while also potentially saving a lot of money.[6]
In fact, with the utility of BBNs as decision support tools that help define variable relationships, outcomes and probabilities of events occurring, plugins have been created in GIS tools to allow BBN applications. As an example, PMAT (Probabilistic Map Algebra Tool) is one such tool developed for QGIS.[7] More commonly, software such as Netica, which are dedicated Bayesian network tools, are applied, where spatial data can become part of the input or exported to common platforms such as ArcGIS for use.[8]
Overall, we see that BBNs have been traditionally narrowly applied to environmental-related topics. However, this is beginning to change, as more risk management and risk-appraising approaches in other areas have realized their potential. Generally, researchers apply both GIS-based and non GIS software to assess spatial BBNs, where the focus is to use BBNs as decision aides. This includes defining important variable relationships and effects.
References
[1] For more on BBNs, see: Pourret, O. (2008) Bayesian networks: a practical guide to applications. [Online]. Chichester, Wiley.
[2] As an example of a decision aid, BBNs are used in ecosystem services applications. For more details, see: Gonzalez-Redin, J., Luque, S., Poggio, L., Smith, R., et al. (2016) Spatial Bayesian belief networks as a planning decision tool for mapping ecosystem services trade-offs on forested landscapes. Environmental Research. [Online] 144, 15–26.
[3] For more on this conservation study on the Julia Creek dunnart, see: Smith, C.S., Howes, A.L., Price, B. & McAlpine, C.A. (2007) Using a Bayesian belief network to predict suitable habitat of an endangered mammal – The Julia Creek dunnart (Sminthopsis douglasi). Biological Conservation. [Online] 139 (3–4), 333–347. Available from: doi:10.1016/j.biocon.2007.06.025.
[4] For more on this study on hydropower licensing and using BBNs, see: Barton, D.N., Bakken, T.H. & Madsen, A.L. (2016) Using a Bayesian belief network to diagnose significant adverse effect of the EU Water Framework Directive on hydropower production in Norway. Journal of Applied Water Engineering and Research. [Online] 4 (1), 11–24. Available from: doi:10.1080/23249676.2016.1178081.
[5] For a review on BBN approaches in ecosystem services, see: Landuyt, D., Broekx, S., D’hondt, R., Engelen, G., et al. (2013) A review of Bayesian belief networks in ecosystem service modelling. Environmental Modelling & Software. [Online] 46, 1–11.
[6] For more on the water mains study, see: Kabir, G., Tesfamariam, S., Francisque, A. & Sadiq, R. (2015) Evaluating risk of water mains failure using a Bayesian belief network model. European Journal of Operational Research. [Online] 240 (1), 220–234.
[7] For more on PMAT, see: https://biblio.ugent.be/publication/6891509/file/6891521.pdf
[8] For an example using Netica, see: Stelzenmüller, V., Lee, J., Garnacho, E. & Rogers, S.I. (2010) Assessment of a Bayesian Belief Network–GIS framework as a practical tool to support marine planning. Marine Pollution Bulletin. [Online] 60 (10), 1743–1754.