The use of GIS is becoming of paramount importance in creating infrastructure for electric cars to become more feasible for more people traveling on road. For one, unlike normal vehicles, simply mapping the shortest or fastest route to a destination might not be feasible for an electric car, as it may need to recharge along the way. Powering stations often need to be incorporated into the calculation, including those that are available and can charge relatively quickly. This make the use of real time data about given powering points, traffic patterns, and road conditions critical. ArcGIS provides some free data on charging stations that help navigate areas. The University of California Davis created an application called EV Explorer that allows you to map your journey using information about charging points and costs of charging in planning a journey.[1] The Centre of Advanced Research in Electrified Transport created a useful application that allows users to locate not only charging point locations and which points charge faster, but real time social media within the application allow users to indicate if a particular point is fully occupied or not.[2]
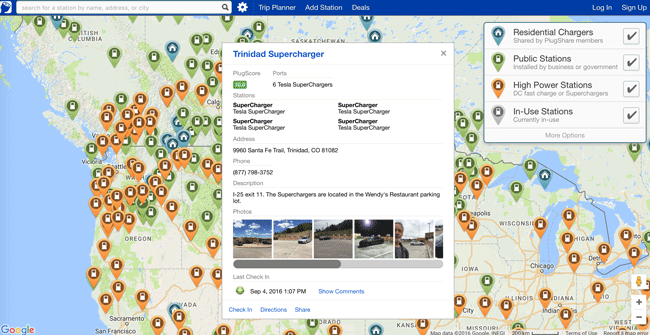
Another aspect of electric vehicles is planning to improve given infrastructure. This requires spatial modeling approaches that integrate factors on likely users of electric vehicles and locations that can be utilized for charging points. Lifestyle, age group, and socio-economic circumstances play a major role on who will adopt such vehicles, affecting where to plan locations for charging stations. The use of census data within spatial regression models becomes useful given trends on who is likely to purchase electric vehicles.[3] Another methodology uses particle swarm optimization that integrates infrastructure costs, construction costs, power supply, and traffic patterns. This simply takes a population of candidate solutions, iterates through them, sees how well given results match given parameters, and then attempts another solution in the next iteration to see if the given set of results create a better fit to empirical data.[4] Other considerations include power distribution in areas where high demand might emerge due to car recharging. Planning models have taken a forecasting approach to look at power variations if different areas are developed into charging stations and likely patterns of traffic affecting them.[5] Such tools and methods are helping drivers navigate access to charging stations, while helping to plan the next generation of charging stations for electric vehicles.
Reference
[1] For more on EV Explorer, see: http://gis.its.ucdavis.edu/evexplorer/#!/locations/start.
[2] For more information on this application, see: http://www.amucaret.com/app-for-real-time-xev-charging/.
[3] For more on adopters of electric vehicles, see: Namdeo, A., A. Tiwary, and R. Dziurla. 2014. “Spatial Planning of Public Charging Points Using Multi-Dimensional Analysis of Early Adopters of Electric Vehicles for a City Region.” Technological Forecasting and Social Change 89 (November): 188–200.
[4] For more on particle swarm optimization used for mapping electric car charging point infrastructure, see: Institute of Electrical and Electronics Engineers, and Power & Energy Society, eds. 2012. IEEE Innovative Smart Grid Technologies – Asia (ISGT Asia 2012): [conference] ; Tianjin, China, 21 – 24 May 2012. Piscataway, NJ: IEEE.
[5] For more on charging stations and power supply demand modeling, see: Wang, Guibin, Zhao Xu, Fushuan Wen, and Kit Po Wong. 2013. “Traffic-Constrained Multiobjective Planning of Electric-Vehicle Charging Stations.” IEEE Transactions on Power Delivery 28 (4): 2363–72.