With winter approaching, one concern that reemerges every year is the occurrence of avalanches that can cause large infrastructural damage and loss of life.
GIS and computational specialists have been developing models and methods to better forecast the likelihood of avalanches, including integrating machine learning techniques, while others have used spatial data to understand behavioral choices and avalanche risk.
Spatial Methods For Forecasting Avalanches
One recent approach developed uses an ensemble modeling approach that combines statistical and machine learning techniques to develop susceptibility estimates.
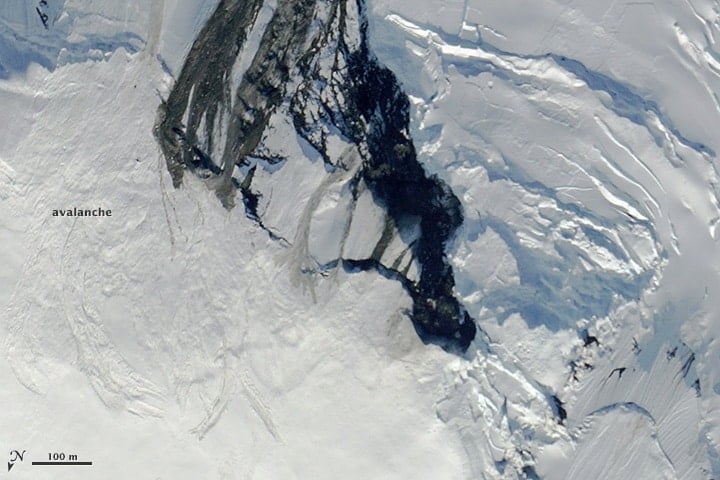
The forecasting capabilities of each model combines to create a more dependable forecast that an area may have a forthcoming avalanche. The approach combines belief function (Bel) and probability density (PD) methods with learning models that incorporate multi-layer perceptron (MLP) and logistic regression (LR).
Imagery is then used to create the training set, incorporating 70 potential avalanche regions, and 30 places that are used to validate results. The weighting derived from the Bel and PD techniques are combined with the MLP and LR learning models to generate a susceptibility map for regions.
In all cases, over 90% accuracy was achieved in estimating the susceptibility of given areas to avalanches using historical data.[1]
While such a technique can be useful for susceptibility measures, one issue local safety experts and managers have to address is when an avalanche is likely to occur based on current environmental conditions.
One approach developed an artificial neural network (ANN) technique that looks at climate data over a 24 year period to train the machine learning model and then estimate the likelihood an avalanche would occur based on hardness, shear strength, temperature, density, thickness and settlement of snowpack layers in a given region.
These conditions are determined to be the most significance factors leading to an avalanche.
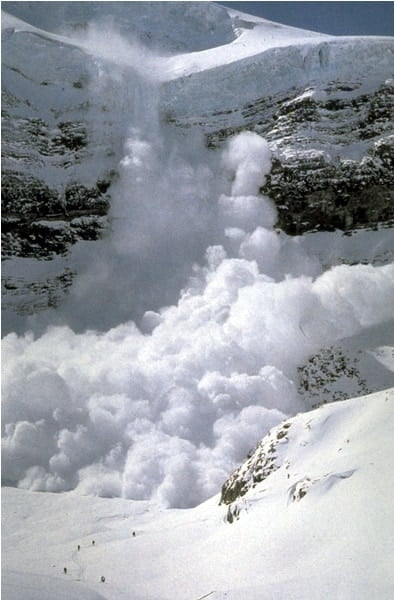
From this approach that integrates these data, and testing using historical data, forecasting the occurrence of avalanches were found to be reasonably good, with the agreement score at around 0.8, showing good forecasting capabilities in timing estimates for avalanches with the factors utilized.[2]
Improved Mapping of Terrain
Other approaches have attempted to improve mapping techniques of terrain.
While elevation is certainly a critical factor in avalanches, presence of snowpack and trees and other obstacles can significantly affect the flow and strength of avalanches. This is why terrain models that capture terrain more accurately at a given, and not just indicate elevation, are needed.
The Avalanche Terrain Exposure Scale (ATES) has been developed as a method to do this, although traditionally such mapping was done by classification based on an individual’s expertise.
One technique recently develop a method to automate the classification for terrain, where they were able to do this at a 10-m resolution for Norway. Such a map has proven to be as reliable as the non-automated version of ATES.
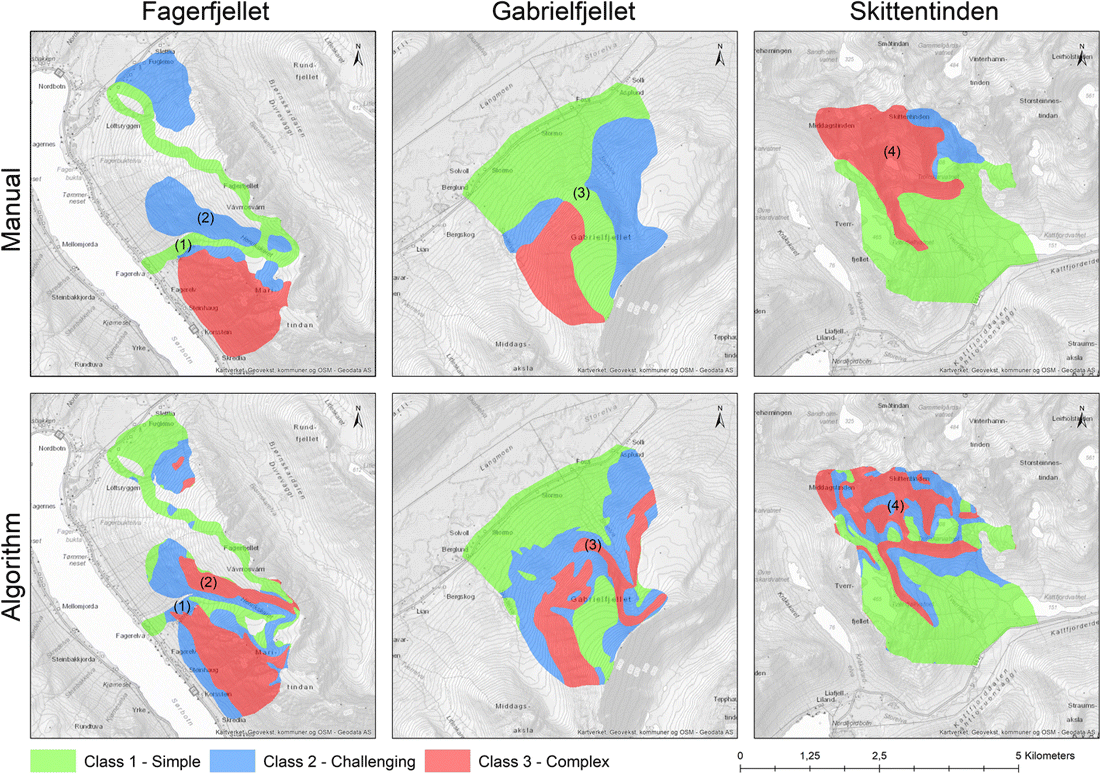
This demonstrates that it is possible to get up-to-date elevation and imagery and combine the information to create an automated risk assessment map for avalanches.[3]
Using GPS Data to Analyze Skier Behavior and Avalanche Risk
Other research has focused on mitigation of avalanches by better learning about human behavior that puts people at greater risk.
This was done by using GPS data from skiers to monitor their behavior choices in areas that faced potential avalanche risk. From this study, it was determined that gender, having some formal avalanche education, and perception of avalanche mitigation applied in a given area affected skier behavior in making less or more risky decisions about approaching areas with potential avalanche risk.
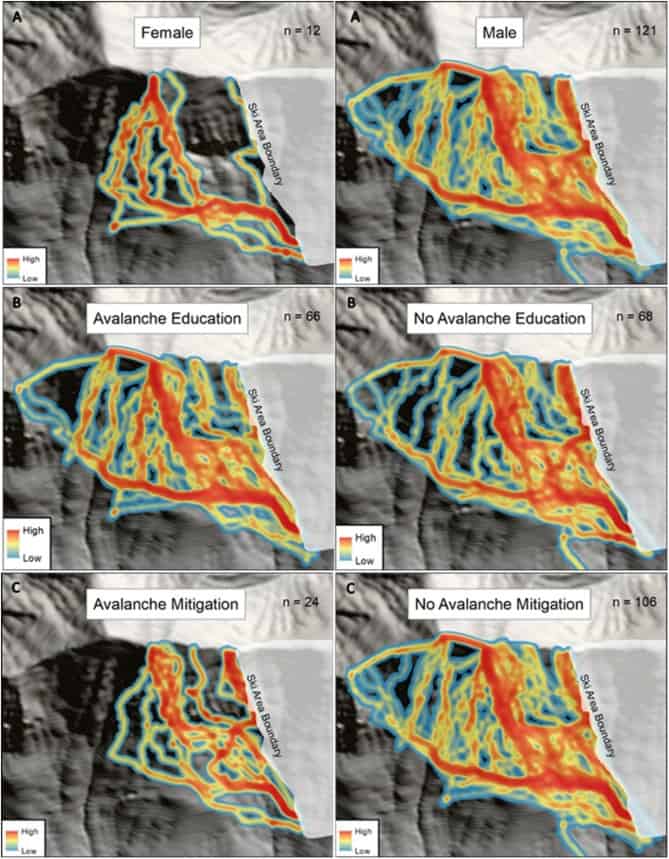
This demonstrates that educating skiers, particularly high risk ones, may also help reduce potential harm for skiers and other recreational users in regions with high avalanche risk.[4]
Modeling avalanches, whether it is determining if a region is prone to avalanches or if given conditions are likely to trigger an avalanche, is increasingly using machine learning techniques within spatial methods.
Using historical climate and snow data, as well as elevation and imagery data, help to determine potential risks in areas.
Other approaches have studied human behavior choices to show that mitigation strategies can also focus on awareness training for higher risk groups in particular.
References
[1] For more on the method that combines statistical and machine learning techniques to estimate susceptibility of areas for avalanches, see: Yariyan, P., Avand, M., Abbaspour, R.A., Karami, M., Tiefenbacher, J.P., 2020. GIS-based spatial modeling of snow avalanches using four novel ensemble models. Science of The Total Environment 745, 141008. https://doi.org/10.1016/j.scitotenv.2020.141008.
[2] For more on the ANN technique for forecasting avalanches, see: Joshi, J.C., Kaur, P., Kumar, B., Singh, A., Satyawali, P.K., 2020. HIM-STRAT: a neural network-based model for snow cover simulation and avalanche hazard prediction over North-West Himalaya. Natural Hazards 103, 1239–1260. https://doi.org/10.1007/s11069-020-04032-6.
[3] For more on mapping using ATES for determining avalanche potential, see: Larsen, H.T., Hendrikx, J., Slåtten, M.S., Engeset, R.V., 2020. Developing nationwide avalanche terrain maps for Norway. Natural Hazards 103, 2829–2847. https://doi.org/10.1007/s11069-020-04104-7.
[4] For more on behavioral choices using GPS tracked data that create risk assessment for individuals in avalanche prone regions, see: Sykes, J., Hendrikx, J., Johnson, J., Birkeland, K.W., 2020. Combining GPS tracking and survey data to better understand travel behavior of out-of-bounds skiers. Applied Geography 122, 102261. https://doi.org/10.1016/j.apgeog.2020.102261.