Proportional hazard modeling has been around for a long-time as a methodology of probability modeling in the social, environmental, medical, and physical science modeling communities. The methodology mostly looks at the probability that given hazards or benefits, as the opposite phenomenon of hazards, may occur for a given occurrence. Traditionally, these models were not oriental spatial, where hazards were often seen as related to a set of factors that were non-spatial. More recently, traditional models have increasingly incorporated spatial phenomenon and relationship affecting hazard functions. One example is the Cox Regression Model, which incorporates elements of time for a given hazard rate and probability for an event to occur. However, additional factors mitigated through spatial effects, such as distance, terrain, or characteristics of a given place, are now increasingly used in this form of modeling. A recent paper looked at fire hazard probabilities across forested regions using a spatially oriented proportional hazard model.[1] Another paper looked at the rise of an empire as a form of spatial hazard model using Cox Regression modeling.[2]
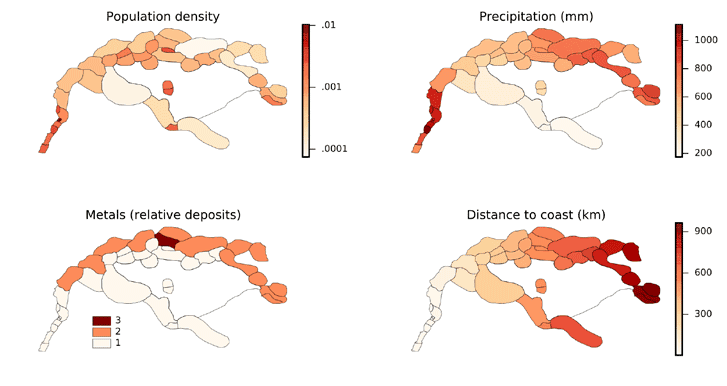
The benefit of these models is they are relatively simple based on probabilistic modeling of given locations and the likelihood events, or hazards, may occur in them. Unlike agent-based models (ABMs), they generally require less data and mostly focus on an overview understanding of given spaces and events. Proportional Hazard Models can be used to understand how given phenomenon emerges as circumstances change, for instance new political or ecological developments in a region could have a chain reaction event of changing probabilities of given spaces. By integrating GIS with this form of modeling, we are now beginning to see GIS increasing its analytical modeling repertoire for the sciences that leverage factors of spatial and time to better understand how emergence and evolution of given processes develops, even when uncertainty is persistent for areas of research. This make GIS and this form of modeling a powerful technique for problems with higher degrees of uncertainty, but where enough is known to measure how given outcomes could emerge under various probability scenarios.
References
[1] For more information about this proportional hazard model, see: Cyr, D., Gauthier, S., & Bergeron, Y. (2007). Scale-dependent determinants of heterogeneity in fire frequency in a coniferous boreal forest of eastern Canada. Landscape Ecology, 22(9), 1325–1339.
[2] For information about the paper dealing with the rise of an empire, see: Baudains, P; Zamazalová, S; Altaweel, M; Wilson, A; (2015) Modeling Strategic Decisions in the Formation of the Early Neo-Assyrian Empire. Cliodynamics: The Journal of Quantitative History and Cultural Evolution , 6 (1), 1 – 23