While we keep seeing signs that electric cars will soon be the standard car, what worries some planners is the ability for electric systems to handle expected increased loads on power infrastructures around the world. GIS and spatial analysis may help find some practical solutions for this problem.
The biggest problem with electric vehicles is the fact they require charging but are mobile, making it more complex than simply expanding networks in a given area. In particular, DC chargers are rapid chargers that can recharge cars within 20 minutes, but require a lot of energy. Furthermore, there are unequal rates with their adoption, where some urban areas are experiencing mass uptake of electric vehicles, while other areas are not. Optimizing electrical car charge points allows both customers to benefit from charging while not overloading the electric system.
Using GIS-based Fuzzy Multi-Criteria Decision Analysis
One work by researchers interested in this problem has assessed electric cars on recharge networks using a method applying fuzzy analytical hierarchy process, where they looked at 15 criteria, in order to determine what locations might be the best to increase power supply. The technique assesses the criteria and weighs their relevance to the power grid. From this, locations that optimize customer needs but balances electric grid capabilities is determined.[1]
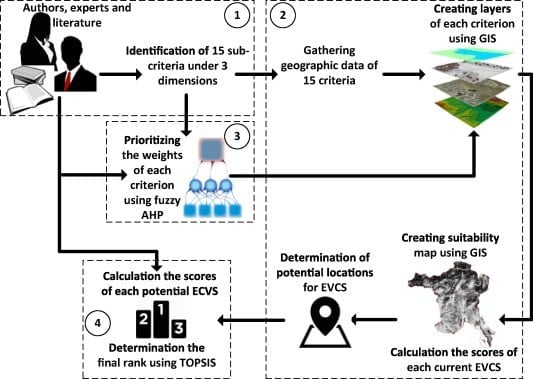
Spatial Load Forecasting Using GIS
Other approaches take a more social-driven perspective. For instance, the adoption of electric cars happens often through word of mouth or influence of people on their social network. Spatial diffusion theory can be used to assess potential areas that are relatively early adopters of electric vehicles and the potential for the spread of adoption in given areas, similar to modeling the spread of rumors in a social network, where adoption of electric vehicles biases demand for electric distribution for given regions. Deterministic modeling in spatial load forecasting estimates the spread of electric vehicle uptake, including its diffusion across areas, in order to forecast electric demand characteristics over time.[2] Other approaches have taken the approach of modeling in detail electrical grid demands and optimization over a fine-scale period using multi-objective optimization, where load demands and power provider limitations, along with distribution of electric cars in a given area are key inputs. These outputs can be checked to determine if real-world data match estimates, which then allows modeling frameworks to be used in forecasting future scenarios.[3]
While many forecasts have assumed electric vehicles as electric consumers, others see the potential that electric cars can add to the electric system. Vehicle to grid technologies enable energy stored in electric cars to go back into the electric system, thereby allowing parked cars, for instance, to be net suppliers, which could help fulfill demand increases. Studies have shown that the wide-scale adoption of such technologies could alleviate demand from large-scale adoption of electric vehicles.[4] Estimates to forecast power demand, therefore, have to consider the number of cars potentially charging and the number of cars giving power to the electric gird. Approaches have, therefore, taken into consideration travel patterns and charging demand, where methods have included using Monte Carlo spatiotemporal simulations, which also consider driver behavior, types of available chargers, and likely distances traveled.[5]
Forecasting electric power needs in light of increased demand based on expected wide-scale adoption of electric vehicles has been considered by a variety of analysts and government officials. This has not discouraged the adoption of these types of vehicles but it has created a variety of methods and analytical approaches to forecasting likely power needs. New technologies, such as electric vehicles giving power back to the electric system and smart grid technologies, means that we are in a better position to meet demand. However, wide adoption of electric vehicles means that infrastructure planning will have to use spatial analysis and a variety of methods that account for decision-making and electric power constraints in future forecasting for electricity needs.
References
[1] For more information on using a fuzzy analytical hierarchy in weighing factors that optimize a given electric grid network for electric cars, see: Erbaş, M., Kabak, M., Özceylan, E., Çetinkaya, C., 2018. Optimal siting of electric vehicle charging stations: A GIS-based fuzzy Multi-Criteria Decision Analysis. Energy 163, 1017–1031. https://doi.org/10.1016/j.energy.2018.08.140.
[2] For more on diffusion theory and how it can be used to forecast the effect of electric vehicles being used in given areas, see: Heymann, F., Pereira, C., Miranda, V., Soares, F.J., 2017. Spatial load forecasting of electric vehicle charging using GIS and diffusion theory, in: 2017 IEEE PES Innovative Smart Grid Technologies Conference Europe (ISGT-Europe). Presented at the 2017 IEEE PES Innovative Smart Grid Technologies Conference Europe (ISGT-Europe), IEEE, Torino, pp. 1–6. https://doi.org/10.1109/ISGTEurope.2017.8260172.
[3] For more on power grid forecasting using multi-objective optimization, see: Einaddin, A.H., Yazdankhah, A.S., 2020. A novel approach for multi-objective optimal scheduling of large-scale EV fleets in a smart distribution grid considering realistic and stochastic modeling framework. International Journal of Electrical Power & Energy Systems 117, 105617. https://doi.org/10.1016/j.ijepes.2019.105617.
[4] For more on vehicle to grid energy supply used to alleviating power supply needs, see: Lim, K., Kim, J.J., Lee, J., 2020. Forecasting the future scale of vehicle to grid technology for electric vehicles and its economic value as future electric energy source: The case of South Korea. Energy & Environment 0958305X1989828. https://doi.org/10.1177/0958305X19898283.
[5] For more on Monte Carlo simulations of electric car recharging demand and electric input, see: Anand, M.P., Bagen, B., Rajapakse, A., 2020. Probabilistic reliability evaluation of distribution systems considering the spatial and temporal distribution of electric vehicles. International Journal of Electrical Power & Energy Systems 117, 105609. https://doi.org/10.1016/j.ijepes.2019.105609