Costs of business are generally one of the biggest burdens any company faces, particularly if there are uncertainties of how much can a business profit from its place.
Integrating GIS for small businesses is today a common phenomenon. Convenience stores are a classical example of small businesses that heavily depend on their locations.
Geomarketing
Retail modeling and gravity flow modeling are two approaches that have been utilized for many decades to assess the benefit of one location relative to others, in particular how likely an area is able to attract people because it is more accessible and beneficial or convenient.
More recent forms of large-scale data analysis utilize more available database information of customers and store information, including purchase patterns and customer volume in given stores at different store times, to use regression models to forecast profitability and expenses.[1]
Using spatial analysis to understand retail customers
More recent approaches integrate collected store data and spatial pattern data from customers over a given time and then apply simulation and modeling techniques, specifically spatial interaction modeling, to then forecast the net benefit or impact of store location on customer behavior. This can allow estimates of profits relative to costs for maintaining and operating retail stores.[2]
In other small retail environments, particular shopping malls, the crowding of many stores make analysis complicated. The use of kernel density estimation, applying ArcGIS Model Builder, has been used to estimate retail success in crowded retail category concentrations. In other words, monitoring competition and customer demand within the clumping of similar stores are critical factors in forecasting retail capability.[3]
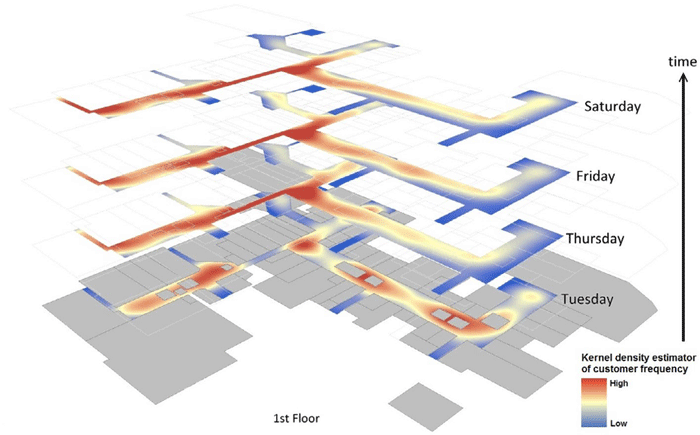
Networking pathways of how stores are accessed and applying Monte Carlo simulation to randomize where stores are located, with different tests looking at clumping or clustering of stores to determine how the location and layout of stores would be more or less successful under given customer conditions, is another area of recent research.
References
[1] For more information on spatial forecasting of retail store locations, see: Wood, S and Browne, S (2007) Convenience store location planning and forecasting – A practical research agenda International Journal of Retail and Distribution Management, 35 (4). pp. 233-255.
[2] For more on store data collection and modeling, see: Merino, María, and Adrian Ramirez-Nafarrate. 2016. “Estimation of Retail Sales under Competitive Location in Mexico.” Journal of Business Research 69 (2): 445–51.
[3] For more on a recent study using kernel density analysis, see: Hirsch, Jens, Matthias Segerer, Kurt Klein, and Thomas Wiegelmann. 2016. “The Analysis of Customer Density, Tenant Placement and Coupling inside a Shopping Centre with GIS.” Journal of Property Research 33 (1): 37–63. doi:10.1080/09599916.2015.1135977.