As awareness of mental health and physical well-being grows in response to the rise in diet-related health concerns, public green spaces are garnering increased attention from both policymakers and the general population.
While this attention is welcome, there is also a need to map these spaces so it is clear who has access to needed spaces. Knowing who has access to such spaces is important so that benefits of green spaces simply do not reflect the various social-economic inequalities in our societies.
In many countries, including developed countries, these spaces are often not distinguished from private or inaccessible green spaces. There are statistical and deep learning methods that can help us better map these spaces using satellite imagery and open data.
Mapping private versus public green spaces
While imagery from satellites such as Sentinel-2 could be used to map green spaces, the main problem is identifying what is public versus private.
Using OpenStreetMap (OSM) and Sentinel-2 data together may prove useful for urban planners who want to distinguish public versus private green spaces. In a relatively recent published method, researchers can map public places by first determining land use polygons that can be derived by simply using OSM data.
Combining satellite imagery with OpenStreetMap data
While OpenStreetMap (OSM) data can provide insights into public access by examining connections between spaces and streets, Sentinel-2 imagery is used to assess the ‘greenness’ of a specific area based on visual data.
In the approach, OSM data and Sentinel-2 satellite imagery are fused, and green spaces are determined through a probabilistic Dempster–Shafer theory. This entails calculating how green something is from imagery using normalized difference vegetation index (NDVI) and then applying Dempster-Shafer to determine the likelihood that a given area was truly green space.
This is in part done to remove the uncertainty from the spatial resolution and values that are sometimes in between classifying something as green or not.
Subsequently, the OpenStreetMap (OSM) tag data, which indicates whether an area is ‘green,’ is utilized to refine the results, allowing for more accurate classification of land as ‘green.
Using OpenStreetMap indicators to determine if a green space is public
Even though this helps determine a green space, the results also need to classify public access and determine if something is truly public. This was done probabilistically using Bayesian logistic regression in classifying if give OSM data indicate public access.

Indicators such as ‘parks’, ‘village green’, or playgrounds would suggest public areas, this was not always the case so a probabilistic model is needed.
In a Bayesian hierarchical approach, if given indicators or tags from the OSM data would suggest public space, then that would mean the space is likely public but there is some probability it would not be classified as such; the model is not strictly deterministic given errors from map data.
The results are also validated using 300 land use polygons selected by hand and compared to the machine-based results.
The final step entails fusing results of green spaces and public access using Dempster-Shafer theory once again. This effectively combined rules that allowed both green and public spaces to be defined and fused in the final classification.
95% overall accuracy in identifying public green space
Overall accuracy did reach 95% when data were combined and checked manually.[1]
This demonstrates that the methodology is fairly accurate but uncertainty remains in places, in part driven by unclear results that can be derived from OSM data given that public spaces are not always clear.
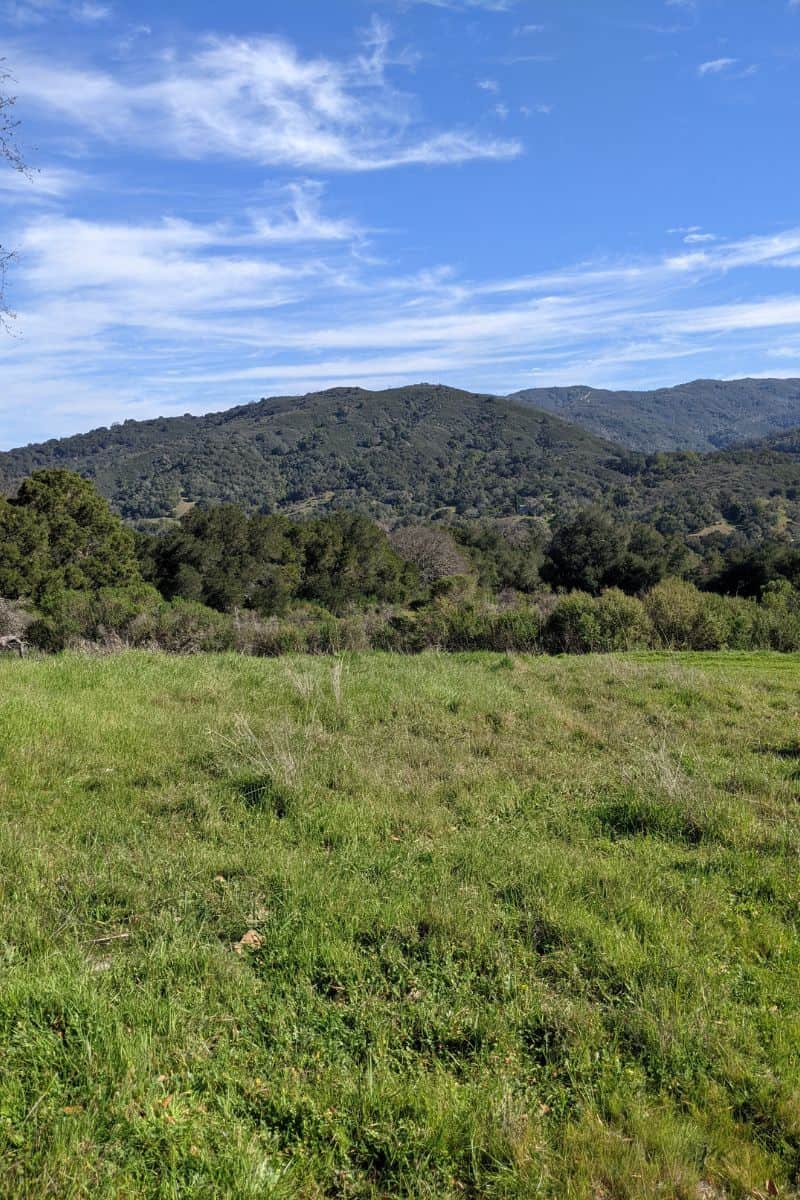
Other approaches to mapping public green space
The approach represents a simpler, perhaps less machine-intensive way in which public green spaces can be determined.
Other approaches have included using deep learning classification, which not only require more data but require training a given model on what a public green space is. One can select from imagery and train a model to know that given areas on imagery would represent a public green space rather than something that was not public.
In this approach, classification from training areas using convolutional neural networks (CNN) helps to determine what is a public green space.
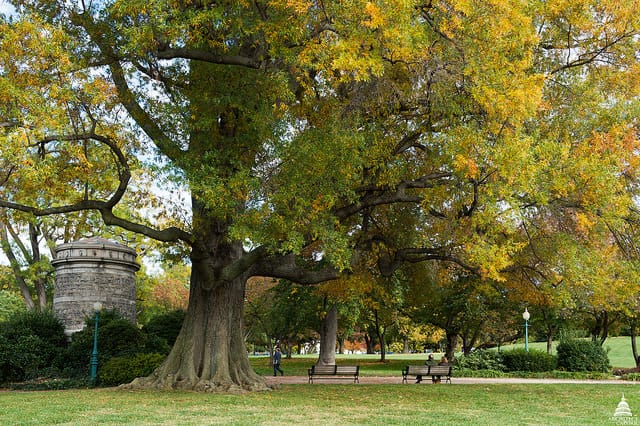
For some parts of the world, this might be difficult without knowing more about given spaces, but the approach is also very accurate, demonstrating levels of accuracy at around 97%.
Such an approach may also require high resolution imagery to capture spaces, given that Sentinel-2’s resolution is 10 m this may not work as well with such imagery.[2]
Statistical and deep learning methods for mapping public green space
What statistical and deep learning approaches demonstrate is that both can be relatively accurate given enough training data or examples. Both methods highlight the fact that imagery and OSM data are needed together given uncertainties in both sets and can help improve accuracy.
More critically, mapping public green spaces will enables better estimates of how much access the wider public, particularly those who live in very urban areas, has to the beneficial qualities of green spaces.
References
[1] For more on using belief function and probabilistic methods to determine public green spaces from satellite imagery and OSM data, see: Ludwig C, Hecht R, Lautenbach S, et al. (2021) Mapping Public Urban Green Spaces Based on OpenStreetMap and Sentinel-2 Imagery Using Belief Functions. ISPRS International Journal of Geo-Information 10(4): 251. DOI: 10.3390/ijgi10040251.
[2] For more on a deep learning approach to mapping public green spaces, see: Huerta RE, Yépez FD, Lozano-García DF, et al. (2021) Mapping Urban Green Spaces at the Metropolitan Level Using Very High Resolution Satellite Imagery and Deep Learning Techniques for Semantic Segmentation. Remote Sensing 13(11): 2031. DOI: 10.3390/rs13112031.