The measurement of vegetation signatures using remote sensing sources has become a critical way to measure the effects of regional and global-scale drought and agricultural production. It is also useful in distinguishing vegetation in regions, including wild and cultivated varieties.
The most common method for this is the normalized difference vegetation index (NDVI) technique.
What is Normalized Difference Vegetation Index (NDVI)?
The concept of normalized difference vegetation index (NDVI) lies with the fact that plants have evolved to reflect near-infrared light, where visible light is strongly absorbed by almost all plants in photosynthesis.
Plants produce pigments in their leaves. This pigment is known as chlorophyll. When sunlight hits an object, some parts of the wavelength are absorbed while other parts are reflected.
Visible light wavelengths range from the shortest (red) to the longest (violet).

When sunlight hits a healthy plant, some of that visible light is absorbed and some of it is reflected. Healthy vegetation absorbs more more red light and blue light and reflects green light which is why leaves look green to the human eye.
The measure of NDVI is thus an index of these two aspects, helping to show how vigorous plant growth is happening in regions. NDVI is a measure of “greenness” or photosynthetic activity in plants
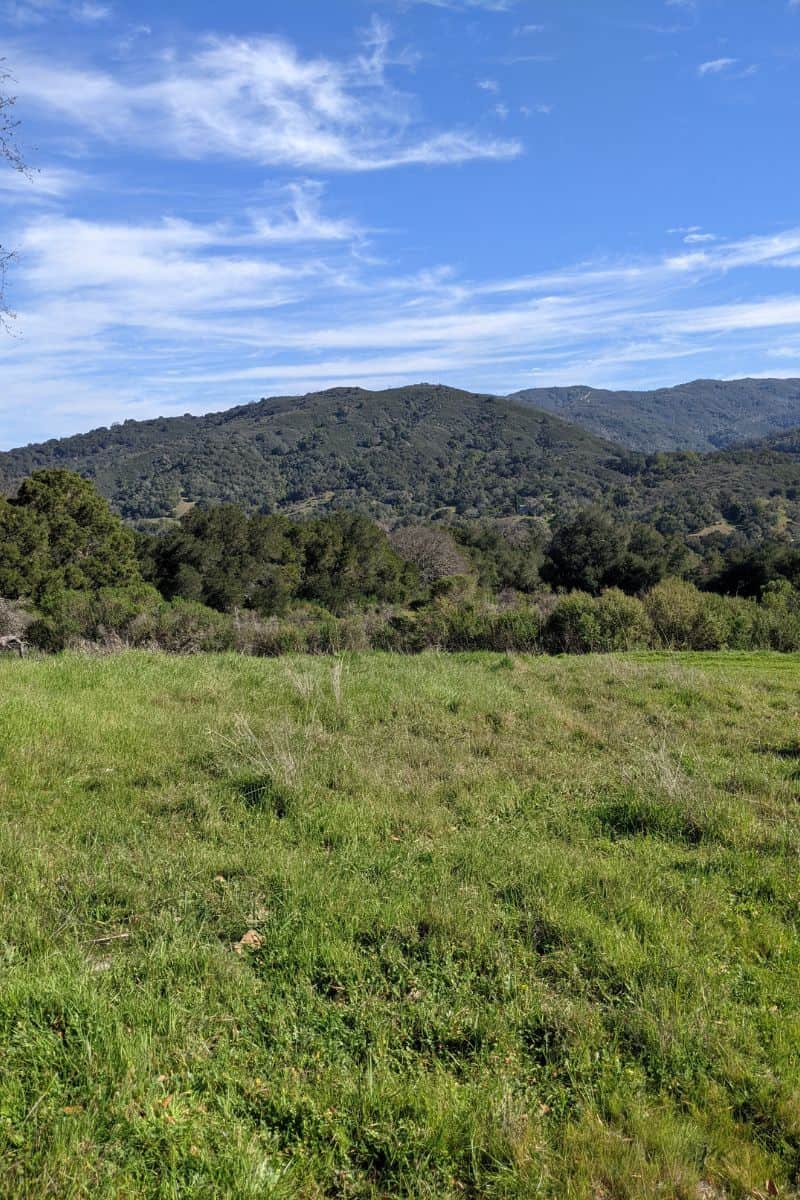
Calibrated results can then be used to estimate vegetation growth and overall biomass, as more vegetation cover will affect the ratio of the different visible light wavelengths absorbed and near-infrared light reflected. The In other words, NDVI looks at the difference in visible and near-infrared reflectance of vegetation. [1]
NDVI can also be used to determine types and sometimes species of vegetation based on reflectance. NDVI can also be used to assess the health of vegetation growth.
Healthier vegetation, which has a higher prevalence of chlorophyll (vegetation greenness) will have a different reflection of near-infrared (NIR) and visible light than stressed or drought-stricken vegetation.
How NDVI values are different between vegetation and non-vegetation areas
NDVI values for vegetation will be positive because healthy vegetation, which is green vegetation, has a high reflectance for near-infrared and a low reflectance for red light.
Areas that have sparse vegetation will have small NDVI values: Low NDVI values show vegetation that is moisture-stressed. Shrubs, grasslands, and meadows will have medium NDVI values. High NDVI values represent more densely populated green areas: tropical forest and temperate forests will have large NDVI values.
NDVI values for water and urban areas will be near zero or negative, this includes areas that are covered by ice, snow, bare rock, soil, or human structures.
Using satellite imagery to measure NDVI
The most common instrument in relation to NDVI has been moderate-resolution imaging spectroradiometer (MODIS), which is on board in the Terra and Aqua satellites. The USGS also produces Landsat Surface Reflectance-derived Normalized Difference Vegetation Index (NDVI) products from several of its Landsat satellites.
Overall, 36 spectral bands are used, giving it a wide range of data capture that covers visible and near-infra red parts of the electromagnetic spectrum.[2]
How NDVI is Used to Understand Vegetation Growth
The method of NDVI has existed since the early 1970s, where today it has become the standard in using multispectral satellite data to measure plant growth.
For instance, in Europe, abandonment and cultivation of farmland has become a major topic of focus. Some abandoned farmland are reused as habitat for native species, while re-cultivation is also occurring as faming benefits are provided to crop lands once abandoned.
Measuring this has policy potential, allowing decision-makers to determine where to focus agricultural efforts while also balancing potentially what areas might be best abandoned or used for conservation purposes.[3]

Other studies have demonstrated that not only overall biomass growth can be measured but biodiversity could be discriminated from the data, while also seasonal changes, such as when flowering occurs and how short-term drought affects some species, could be measured using more frequent intra-seasonal imagery.[4]
Using NDI to Measure Agricultural Yield
One of the biggest areas for NDVI has been measuring agricultural yield, as this allows national and international organizations to assess what yield outputs will be and potentially to monitor if likely areas may experience drought and possible famine due to environmental conditions.
The use of NDVI has been used to forecast in advance of yield production by looking at the stage of development for agricultural vegetation and comparing it to the past. The utility of this allows adequate time for drought-related decisions to be made by relevant agencies and governments.[5]

One challenge has been developing forecasting methods using NDVI that is spatially scalable and temporally consistent. Techniques have been introduced to integrate statistical, imagery, and simulation-based results to predict yields in different regions.
Seasonal variability and different sensitivities to environmental conditions have made forecasting particularly challenging in some countries.
Using multiple techniques, therefore, might be the best option, where techniques could be developed more specifically for countries or regions that have greater chance of environmental variability.[6]
Overall, it is the simplicity of the NDVI technique and its applicability to vegetation-base studies that have helped to make it perhaps the most extensively used in categories of remote sensing techniques used to monitor agriculture and plant growth.
References
[1] For more on the methods of NDVI, see: Shunlin Liang (ed.) (2008) Advances in land remote sensing: system, modelling, inversion and application. S.l., Springer, pg. 186.
[2] For more on MODIS, see: Anon (2012) Remote Sensing of Drought Innovative Monitoring Approaches. CRC Pr I Llc, pg. 69.
[3] For more on this study focusing on using NDVI to measure abandoned and re-cultivated land, see: Estel, S., Kuemmerle, T., Alcántara, C., Levers, C., et al. (2015) Mapping farmland abandonment and recultivation across Europe using MODIS NDVI time series. Remote Sensing of Environment. [Online] 163, 312–325.
[4] For more on the study measuring biodiversity and intra-seasonal variability in a prairie setting, see: Wang, R., Gamon, J., Montgomery, R., Townsend, P., et al. (2016) Seasonal Variation in the NDVI–Species Richness Relationship in a Prairie Grassland Experiment (Cedar Creek). Remote Sensing. [Online] 8 (2), 128.
[5] An example of using NDVI to make predictions can be found here: y, L., Newlands, N., Davidson, A., Zhang, Y., et al. (2014) Assessing the Performance of MODIS NDVI and EVI for Seasonal Crop Yield Forecasting at the Ecodistrict Scale. Remote Sensing. [Online] 6 (10), 10193–10214.
[6] For more on integrating remote sensing data with different mathematical techniques for forecasting yields using NDVI approaches, see: Azzari, G., Jain, M. & Lobell, D.B. (2017) Towards fine resolution global maps of crop yields: Testing multiple methods and satellites in three countries. Remote Sensing of Environment. [Online] Available from: doi:10.1016/j.rse.2017.04.014 [Accessed: 14 July 2017].
Disclaimer: GIS Lounge is a participant in the Amazon Services LLC Associates Program, an affiliate advertising program designed to provide a means for sites to earn advertising fees by advertising and linking to amazon.com.
Advances in Land Remote Sensing, Editor: Shunlin Liang, Springer Netherlands, 2008, 978-1402064494, 497 pp.
Remote Sensing of Drought: Innovative Monitoring Approaches (Drought and Water Crises)
Editors: Brian D. Wardlow, Martha C. Anderson, James P. Verdin. CRC Press, 2012, 978-1439835579, 484 pp.