With growth in the area of social media, many approaches have developed to understand the spatial patterns of social media, such as in Twitter, Yelp, Facebook, and other sites.
One prominent area is topic modeling, which is the study of delineating topics from media content.[1] While topic modeling by itself has been an increasingly growing research field in the humanities and social sciences, where the use of social media and newspapers, in particular, have helped to identify many topical trends, GIS has only recently been employed more prominently in this area.
Trends in Public Health With Topic Modeling and GIS
One example of research in topic modeling and GIS includes understanding trends in public health, in particular obesity, and the types of themes or topics discussed in relation.
Furthermore, visualizing and understanding spatial patterns, which may indicate trending factors that influence public health (e.g., rural vs. urban regions in areas of high obesity) concerns in different areas. Related: Using Satellite Imagery to Map Obesity Rates
Obesity tweets were, for instance, found to primary concentrate in the eastern portion of the United States and in some areas along the coastal states. When looking at the reasons why, researchers suggested that a very strong correlation between location of fast food restaurants and obesity related tweets are evident in data.
In this case, the Latent Dirichlet Allocation algorithm, a type of probabilistic determination of topics commonly used in topic modeling, was used to show the prevalence of topics in regions. Location information was generated from tweets and could then be compared to data on restaurant locations.[2]
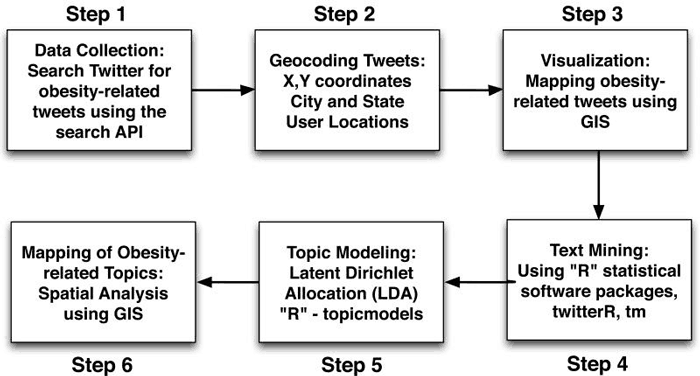
Location Recommendation and Topic Modeling
Outside of academic applications, however, the use of topic modeling has many potential benefits for commerce.
In one recent study, user tweets and use of Yelp was utilized to determine topics of interest, such as restaurants or activities. These interests were correlated with where individuals were.
The spatial and topical patterns were then utilized to create a learning algorithm that can estimate likely interests for individuals in a given area using prior historical use. For instance, if a site or area has restaurants the person might like, given previous topics of interest, then those patterns determined by the topic model could inform which likely restaurant would be of interest.[3]
In effect, it is a type of recommendation algorithm that could be utilized by commercial sites or other applications that can guide people to given places of interest. Because of commercial potential of spatial topical modeling, efforts have also focused on spatiotemporal topic modeling that looks at where individuals might be and, using previous patterns of topic searches or use of social media, then recommendations are made as to what areas or activities might of interest in a surrounding place the individual might likely be in at given times.
Extending this potential, combining spatiotemporal results with profiles of users, such as age, sex, and other aspects, allow the data to be aggregated for larger cohorts so that future recommendations could be made to others with similar profiles.[4]
In effect, this could be done to characterize what others, where less data on them is available, may be interested in when visiting or coming to a location.
Overall, topic modeling shows a lot of commercial and academic potential. It has branched out to other areas with geospatial applications, including in transportation and using topical trends to determine transport research and focus areas.[5]
The focus on spatiotemporal modeling is likely to be a greater focus in the future, given the financial potential. While topic modeling and GIS are still a very nascent area of research, recent papers have shown wide application potential.
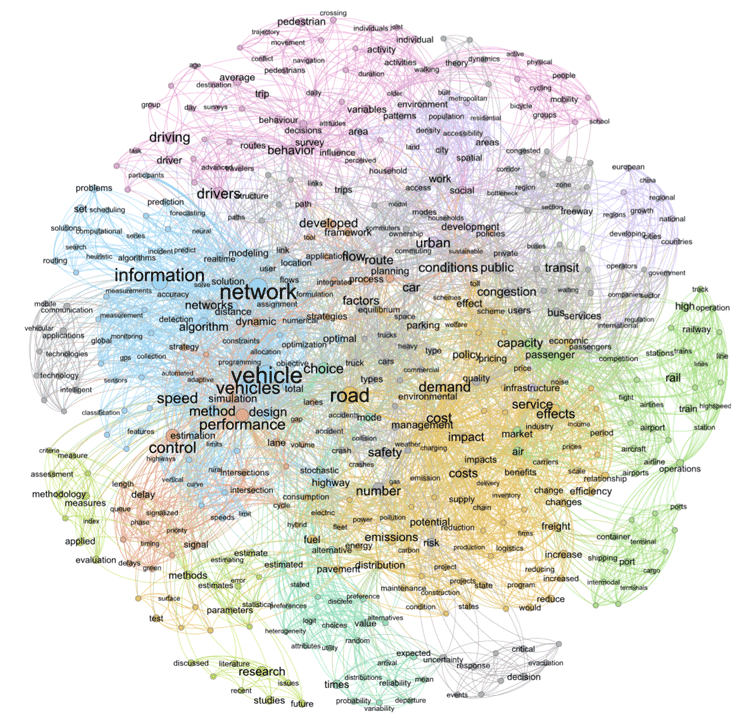
References
[1] For more on topic modeling, see: Landauer, Thomas K., ed. 2007. Handbook of Latent Semantic Analysis. Mahwah, NJ: Erlbaum.
[2] For more on this study that used LDA and spatial methods, see: Ghosh, Debarchana (Debs), and Rajarshi Guha. 2013. “What Are We ‘tweeting’ about Obesity? Mapping Tweets with Topic Modeling and Geographic Information System.” Cartography and Geographic Information Science 40 (2): 90–102.
[3] For more on using topic modeling and past use behavior, including how it can be utilized to guide future interests in given areas, see: Hu, Bo, and Martin Ester. 2013. “Spatial Topic Modeling in Online Social Media for Location Recommendation.” In , 25–32. ACM Press. doi:10.1145/2507157.2507174.
[4] For more on spatiotemporal topic modeling and GIS, see: Hu, Bo, Mohsen Jamali, and Martin Ester. 2013. “Spatio-Temporal Topic Modeling in Mobile Social Media for Location Recommendation.” In , 1073–78. IEEE. doi:10.1109/ICDM.2013.139.
[5] For more on topic modeling and transport, see: Sun, Lijun, and Yafeng Yin. 2017. “Discovering Themes and Trends in Transportation Research Using Topic Modeling.” Transportation Research Part C: Emerging Technologies 77 (April): 49–66. doi:10.1016/j.trc.2017.01.013.