There are many different applications for satellite imagery. Technology has improved to the point where an object in space can detect miniscule changes in data thousands of miles beneath it on the surface of the Earth. From water levels to population density, suburban sprawl to the species of trees growing in individual forests around the world, satellite imagery can map a nearly unlimited amount of data.
Satellite images are now being used to map poverty levels around the world. Tracking poverty can help governments, non-governmental organizations, and researchers, among others, understand where poverty is, how severe it is, and how it can be alleviated. By using satellite imagery the amount of work on the ground is reduced, which also reduces costs and risk factors associated with working in poverty-stricken parts of the world.
Satellites orbiting the Earth take specific pictures that are able to be manipulated to determine different kinds of information. The same picture can be used for a nearly unlimited number of purposes by any organization with access to the satellite images and the ability to analyze the data contained within.
Current poverty data shows that electricity can be used to determine where richer people live as compared to poorer areas. However, the specific details of what kind of poverty people are facing is harder to determine using lights. Researchers at Stanford are using other indicators to determine poverty levels; these indicators include access to water, location in comparison to an urban center and food sources, and if agriculture is viable part of the economy nearby.
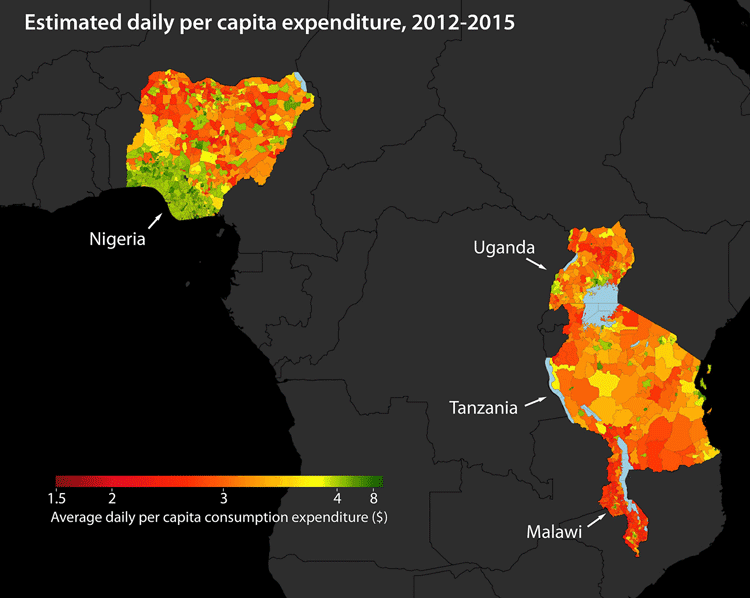
The Stanford team went a step further and created a computer program that can learn as it analyses the satellite imagery. People looking at thousands of images would take a long time, and people may miss some of the important big picture data when they were looking at such small images. The Stanford computer learned to analyze the specific poverty data using a convolutional neural network. They used satellite data from Nigeria, Tanzania, Uganda, Malawi, and Rwanda.
The satellite data proved to be fairly accurate, but ground surveys are still the best way to make sure collected information on poverty remains accurate. Obtaining ground survey information is sometimes impossible in certain locations, which is where computers and satellite data come into effect. Together these information gathering methods are the most accurate, but either can be used in the growing effort to map poverty around the world.
More:
Jean, N., Burke, M., Xie, M., Davis, W. M., Lobell, D. B., & Ermon, S. (2016). Combining satellite imagery and machine learning to predict poverty. Science, 353(6301), 790-794.
Horton, M. (2016, August 18). Stanford scientists combine satellite data, machine learning to map poverty.
